Mechanical behaviors such as elastic, thermoelastic, and viscoelastic textile composites involving multiple scales and complex geometries can be challenging to predict. Mechanics of structure genome (MSG) has been extended to accurately capture the above mechanical behaviors. Compared with traditional representative volume element (RVE) analysis using 3D finite element analysis (FEA), the MSG-based models provide same accuracy at a fraction of computing costs. Taking advantages of the advanced machine learning models such as neural network models, data-driven models have been developed to further accelerate the multiscale modeling of textile composites. The efficient surrogate models have been applied to model failure criteria of yarns, thermal conductivity, and failure strength of textile composites. The developed functions have been implemented into an open source code in the cloud called TexGen4SC, which is freely accessible and can be launched through a web browser (https://cdmhub.org/tools/texgen4sc) without installation.
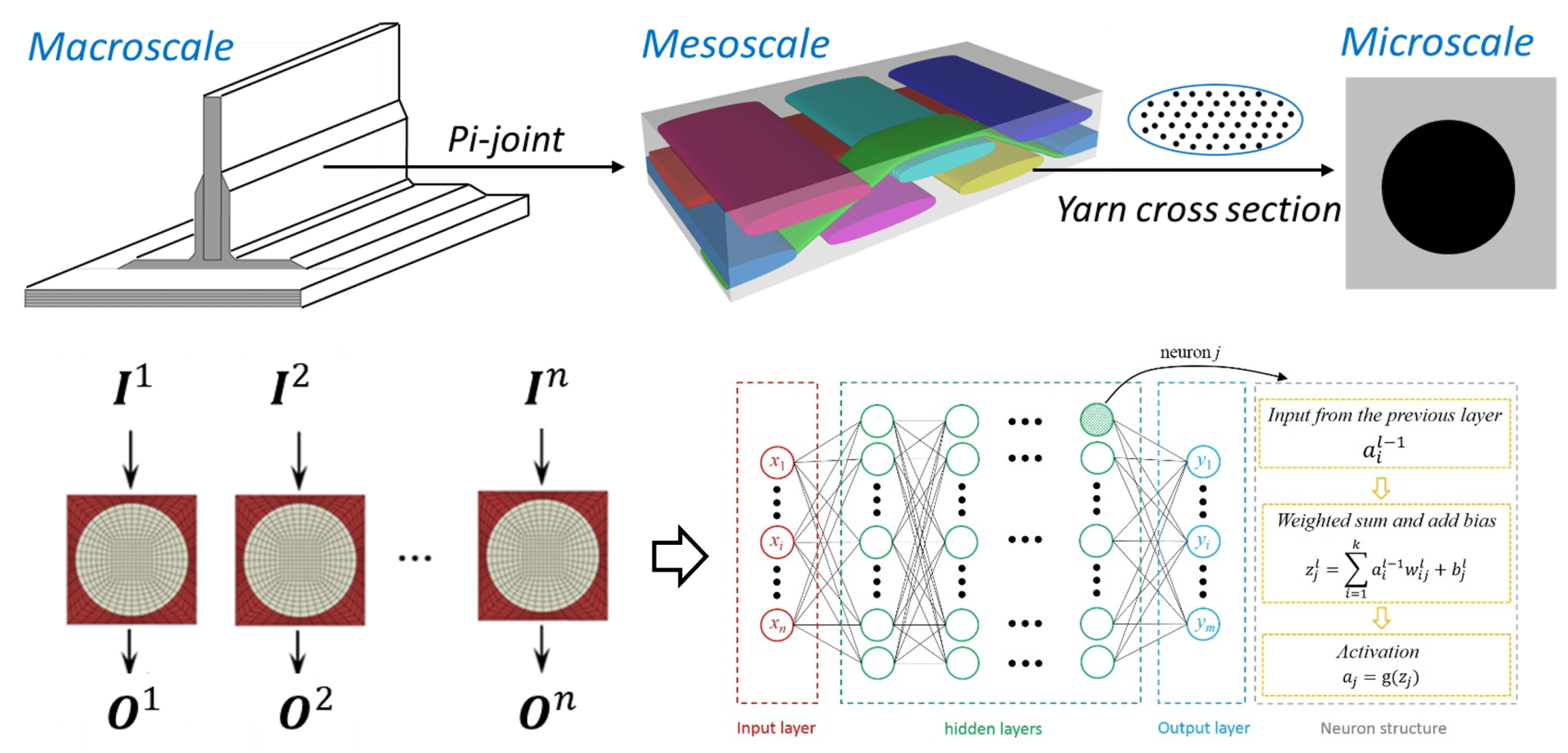
Reference:
- Liu, X., Zhou, X.Y., Liu, B. and Gao, C., 2023. Multiscale modeling of woven composites by deep learning neural networks and its application in design optimization. Composite Structures, p.117553. DOI: 10.1016/j.compstruct.2023.117553.
- Liu, X., Tao, F. and Yu, W., 2021. A review of artificial neural networks in the constitutive modeling of composite materials, Composites Part B: Engineering, 224, p.109152. DOI: 10.1016/j.compositesb.2021.109152.
- Liu, X., Peng, B. and Yu, W., 2021. Multiscale modeling of the effective thermal conductivity of 2D woven composites by mechanics of structure genome and neural networks. International Journal of Heat and Mass Transfer, 179, p.121673. DOI: 10.1016/j.ijheatmasstransfer.2021.121673.
- Rique, O., Liu, X., Yu, W. and Pipes, B., 2020. Constitutive modeling for time- and temperature- dependent behavior of composites, Composites Part B: Engineering, 184, p.107726. DOI: 10.1016/j.compositesb.2019.107726
- Liu, X., Gasco, F., Goodsell, J. and Yu, W., 2019. Initial failure strength prediction of woven composites using a new yarn failure criterion constructed by deep learning. Composite Structures, 230, p.111505. DOI: 10.1016/j.compstruct.2019.111505
- Liu, X., Gasco, F., Yu, W., Goodsell, J. and Rouf, K., 2019. Analyze complex woven composite structures using MSC.Nastran, Advances in Engineering Software, 135, p. 102677. DOI: 10.1016/j.advengsoft.2019.04.008
- Liu, X., Yu, W., Gasco, F. and Goodsell, J., 2019. A unified approach for thermoelastic constitutive modeling of composite structures, Composites Part B: Engineering, 172, pp. 649-659. DOI: 10.1016/j.compositesb.2019.05.083
- Liu, X., Tang, T., Yu, W. and Pipes, B., 2018. Multiscale modeling of viscoelastic behaviors of textile composites, International Journal of Engineering Science, 130, pp. 175-186. DOI: 10.1016/j.ijengsci.2018.06.003
- Rouf, K., Liu, X. and Yu, W., 2018. Multiscale structural analysis of textile composites using mechanics of structure genome, International Journal of Solids and Structures, 136, pp. 89-102. DOI: 10.1016/j.ijsolstr.2017.12.005
- Liu, X., Rouf, K., Peng, B. and Yu, W., 2017. Two-step homogenization of textile composites using mechanics of structure genome, Composite Structures, 171, pp. 252-262. DOI: 10.1016/j.compstruct.2017.03.029